#62: 🦾 Tech Advancements Driving Digital Therapeutics
AI and machine learning are enabling accurate and reliable personalized health interventions
Hi Friends,
Nina here.
Each week I address topics on health, longevity and psychedelics suggested by you.
If you’re not a subscriber, here’s what you missed in November.
Subscribe to get every post delivered straight to your inbox.
💬 In this note:
🦾 Tech Advancements Driving Digital Therapeutics
📚 The Storied Life of A.J. Fikry
⚡️ Nature Photos
🦾 Tech Advancements Driving Digital Therapeutics
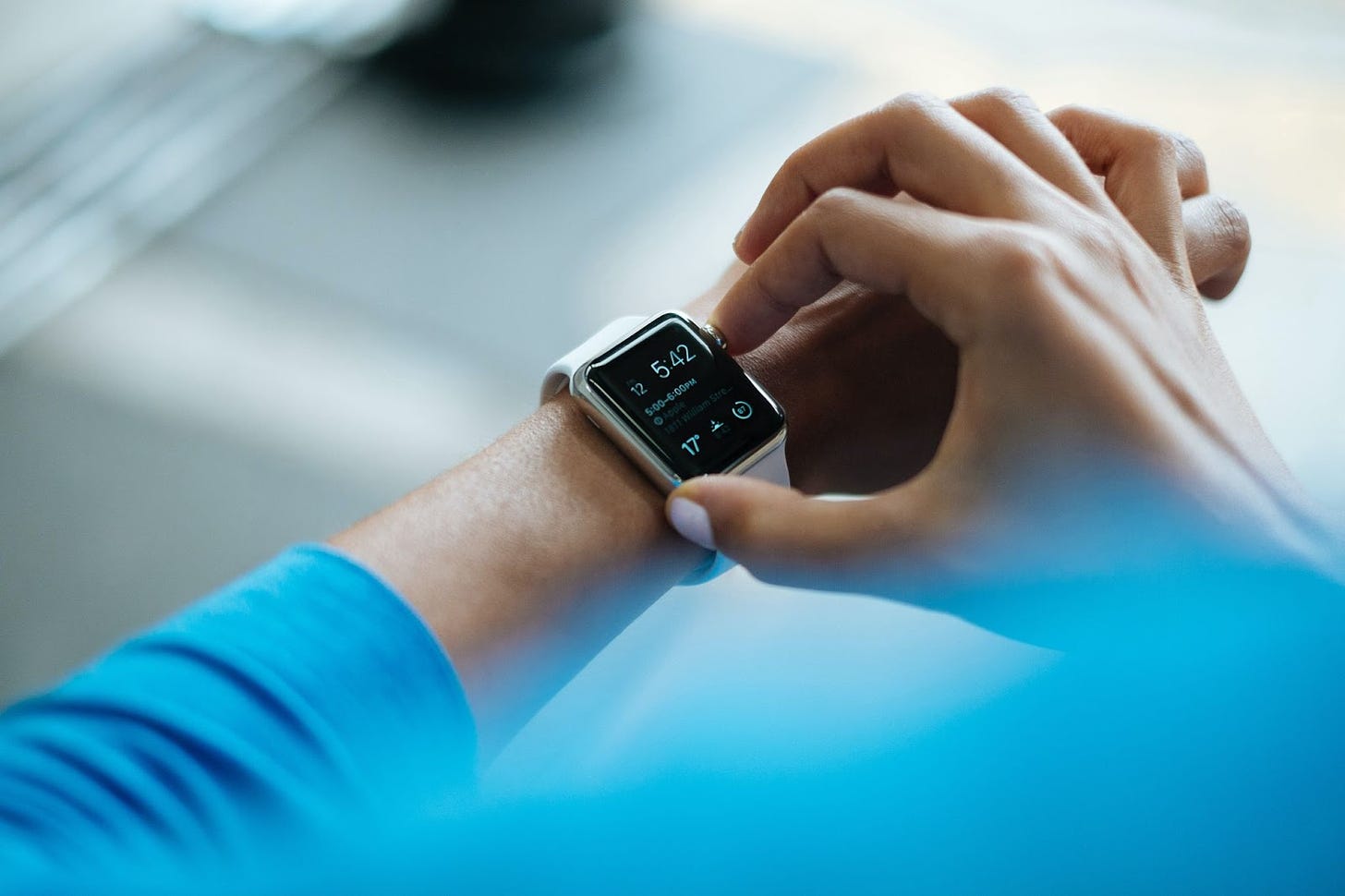
Digital therapeutics are evidence-based treatments delivered through software programs.
There are many digital interventions for health, but not all are actual therapeutics. For a software to be considered a digital therapeutic, it has to go through rigorous regulatory and clinical evaluation to show improvements on a disease.
This flowchart sums it up.
Want more details? Check out Nina’s Notes #51.
DTx have been applied to various medical fields, such as chronic diseases, physical diseases and mental health.
The development of a digital therapeutic requires a multidisciplinary team with a lot of technical support to securely collect data from the patient, then process and analyze the data and finally provide personalized treatment methods to the patient.
This requires the use of cutting-edge computing technologies to integrate big data, artificial intelligence (AI) and machine learning in healthcare.
Technologies driving DTx
To develop and commercialize a DTx, technologies need to be used that fall into four core categories - data collection, data preprocessing, machine learning and federated learning.
Data Collection
Right now, your doctor may use rating scales, questionnaires, medical image data or biosignal data to collect data about your symptoms and the severity of symptoms of a disease.
This data collection can be improved, automated and repeated more frequently with the help of digital technologies.
Many digital therapeutics are currently developed in a mobile application format. Mobile apps can collect additional data through a user’s wearable device allowing for a continuous collection of data in real-time.
Smart watches, bracelets, rings, chest bands and shoe insoles have all been developed to gather human biosignal data.
Within these wearables are photoplethysmogram [photo-pleth-is-mo-gram] (PPG) sensors, gyroscopes, accelerometers, pressure sensors, skin conductivity sensors, electromyography [electro-my-o-graphy]sensors, and even sweat rate sensors.
Wearables are much more than just step counters!
These sensors can measure heart-related data, such as heart-rate, heart rate variability and electrocardiogram.
DTx products can optimize the patient’s data to provide a more objective treatment according to their targeted disorder.
In addition to collecting patient data from mobile devices and wearables, virtual reality (VR) devices have recently been developed to collect disorder-related data.
For example, there is research to identify and determine attention deficit and hyperactivity disorder (ADHD) symptoms in children based on collected VR data.
The study created a virtual world to collect data from patients in different virtual reality scenes. Then the ADHD classification was conducted with an AI model created through a DSM-5-based rating scale.
Data Preprocessing
Data collected from wearable sensors and smart devices is complex and noisy, so it needs to be filtered and processed to be useful for analysis.
When collecting and analyzing data, it is crucial to ensure the data does not contain personal information that could be used to identify individuals. Health data is very sensitive and must be controlled and protected according to data protection regulations such as HIPAA in the United States and GDPR in Europe.
To protect the privacy of the patients and users, data must be collected anonymously or be made anonymous (a process called de-identification) after collection.
Technologies have been developed to make this process easier, faster and unbiased.
Techniques such as pattern-matching de-identification algorithms and recurrent neural network models have been developed to de-identify critical patient information from medical records.
These methods ensure only the necessary data is used for analysis without revealing patient identities.
Privacy measures like these are widely used in medical research to meet international standards and are commonly used for complex data like medical images.
Data Analysis
Performance of DTx requires a high rate of sensitivity and specificity from the data analysis.
In the development stage of a DTx, analysis of the research data is necessary to generate algorithms for diagnosis, classification and treatment recommendations.
Once the DTx is developed and commercialized, patients using the DTx can expect accurate analysis results on their medical condition and personalized treatments based on their unique data.
With the rapid developments in AI, various machine learning techniques can be applied in the DTx development to ensure high accuracy and reproducible results.
Machine learning for DTx
In the past, medical data analysis traditionally relied on statistical analysis techniques. Now the medical AI field is expanding with the development of advanced machine learning algorithms.
These new algorithms are more effective because they can learn and recognize hidden data features that are not obvious to humans.
By training data features through deep neural networks, machine learning can be applied in radiology, pathology, neuroscience, genetics, mental illness and other chronic diseases.
Digital therapeutics use this medical AI technology to diagnose and classify diseases.
For medical imaging data, two machine learning techniques are used.
Convolutional neural networks (CNNs) such as prediction, classification, segmentation and augmentation are used for analyzing medical images to extract useful information and aid decision-making from the original input image.
Recurrent neural networks (RNNs) and transformer-based models are important for analyzing time-series data to monitor changes in a patient's condition.
Machine learning has been applied extensively in medical imaging to help make final diagnostic decisions across many health care conditions and on various images produced by MRIs, CT scans and X-rays.
Federate Learning for DTx
DTx deals with sensitive medical data and machine learning models need to be trained at a single server site or institution.
When using this training method, data leakage or security attacks become possible. These are a very dangerous risk for companies and research institutions when dealing with medical data.
Federate learning (FL) is an alternative structure which allows researchers to train their models without data security concerns, as it doesn't require collecting data from users but rather uses distributed learning across devices.
In FL, a central server generates one initial global machine learning model. This model is sent to the devices participating in the learning procedure. These devices then learn from it using their data and send back updated model weights to the server, thus avoiding the direct sharing of sensitive data.
FL is used in medical AI to meet data protection regulations like GDPR and HIPAA, allowing for secure data analysis without compromising privacy.
Research in FL has shown effective results in areas like pediatric chest radiographs, diagnosing hypertrophic cardiomyopathy, arrhythmia classification, and emotional state analysis, which match the performance of traditional machine learning methods.
While FL offers benefits for digital therapeutics, challenges remain in adapting it to diverse mobile platforms and a wide variety of wearables while still ensuring efficient data processing.
The implementation of FL in digital therapeutics needs further development to address issues like hardware compatibility, client management, and data security.
Advantages of Digital Therapeutics
These advances in machine learning, wearable technology and artificial intelligence are enabling the rapid development of digital therapeutics.
DTx can provide the most optimized treatment for an individual patient based on the patient’s real-time data and medical history.
A personalized treatment design can be developed, managed and tracked through DTx products, rather than relying on infrequent meetings with a doctor and treatment provided at home or in a hospital.
DTx are getting us one step closer to better preventative care and the ultimate goal of totally personalized medicine.
📚 Book of the Week
The Storied Life of A.J. Fikry by Gabrielle Zevin
Rating: ★★★★★
A perfect book lover’s romantic dream to end the year.
A bookshop owner recently lost his wife, his happiness and his will to live. Suddenly his life is changed when a surprise is delivered to his bookshop. Meanwhile, a relationship builds between a city girl and the bookshop owner on a charmingly remote island.
It was a quick read, funny at times and charming. I found myself rooting for the two to get together and enjoyed seeing the maturity of their love.
Also it’s written by Gabrielle Zevin, the genius storyteller behind Tomorrow and Tomorrow and Tomorrow (BOTW November 8, 2023, ★★★★★)
Is it the inspiration for a Hallmark Christmas Classic? No, but it is a movie and it’s cute.
⚡️ Check This Out
Behold the breathtaking photos of the natural world.
The Nature Conservancy’s 2023 photo contest winners.
These are my three favorite photos.
Check out the whole collection here.
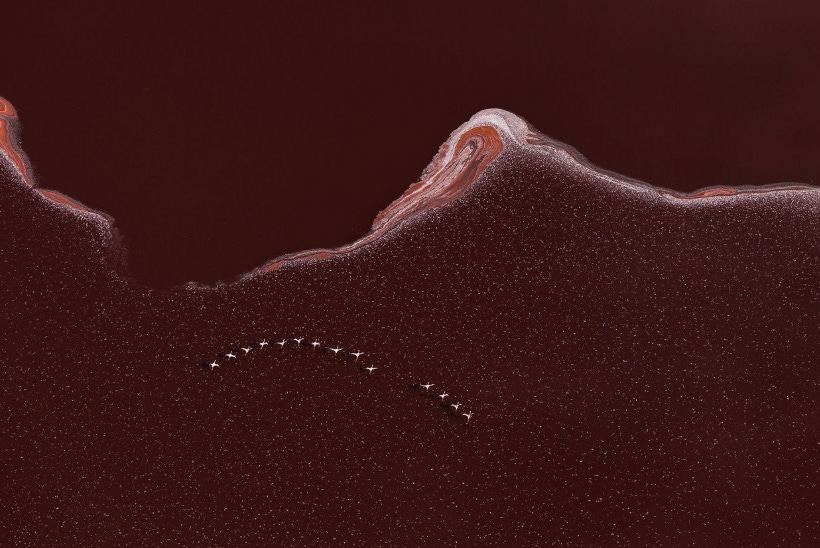
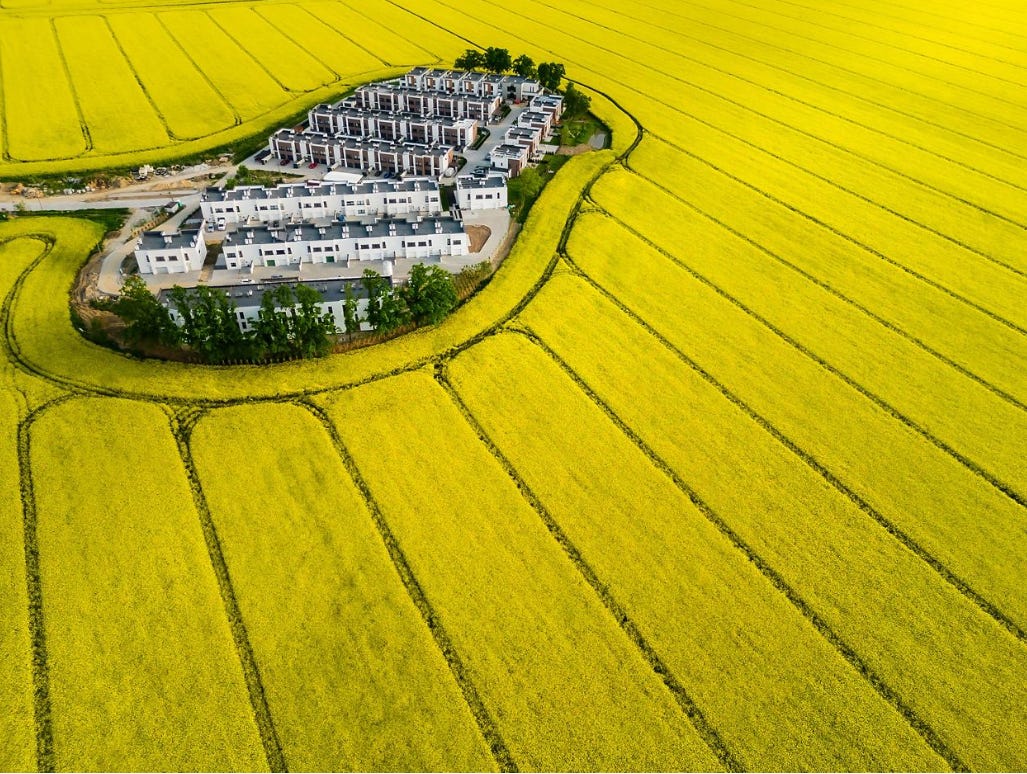
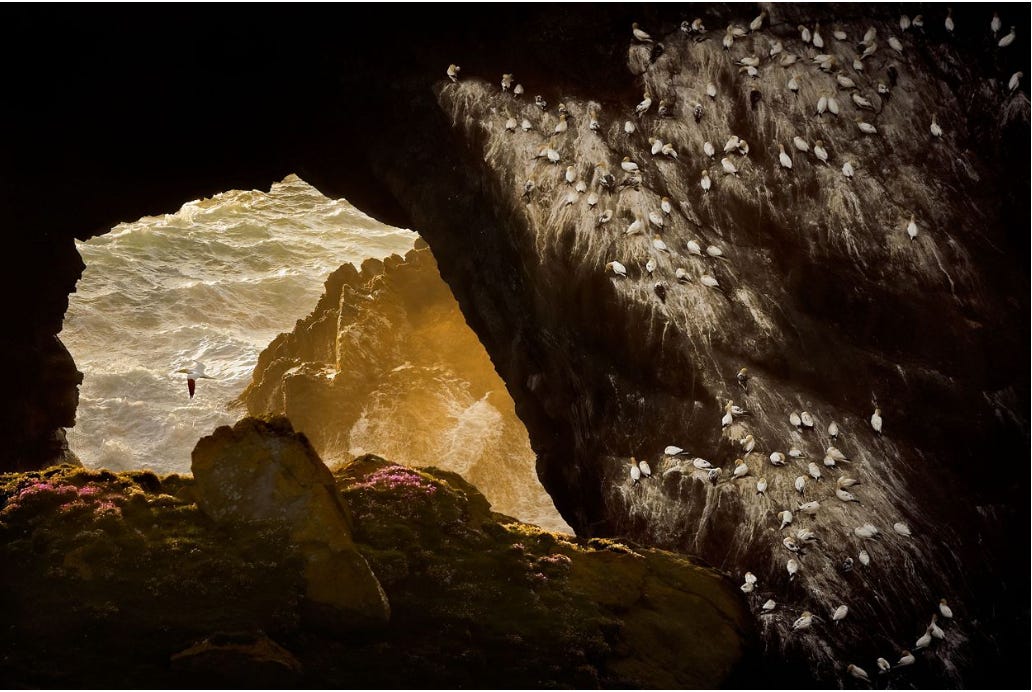
Edited by Wright Time Publishing